Putting it all Together: A Product Analytics Maturity Model
Are you considering integrating Pendo into your organization, or are you in the midst of these challenges? Leveraging Pendo's powerful product analytics can transform the way you understand and engage with user data to make informed decisions. For product leaders and managers, mastering product analytics is imperative to achieve success in today's competitive market. Interested in exploring this together? Let's chat!
We present a Product Analytics Maturity Model that can help lay out a roadmap of evolution for PM's with increasing sophistication in insights generated and presented.
Introduction
This is the 9th post in our series on Product Analytics Integration Best Practices. We've covered a lot of content in the last several weeks, written for Product Managers to help them build up a sophisticated set of analytics reporting that helps them operate as data-informed as possible in their product leadership. Today's post focuses on how PM's should "product manage" their analytics efforts. We present a Product Analytics Maturity Model that can help lay out a roadmap of evolution for PM's with increasing sophistication in insights generated and presented.
This maturity model came about from a Modern Product engagement that involved Pendo integration and product analytics practice development with a rollout to a large client with many agile product teams. The ideas presented here can be applied to any analytics platform.
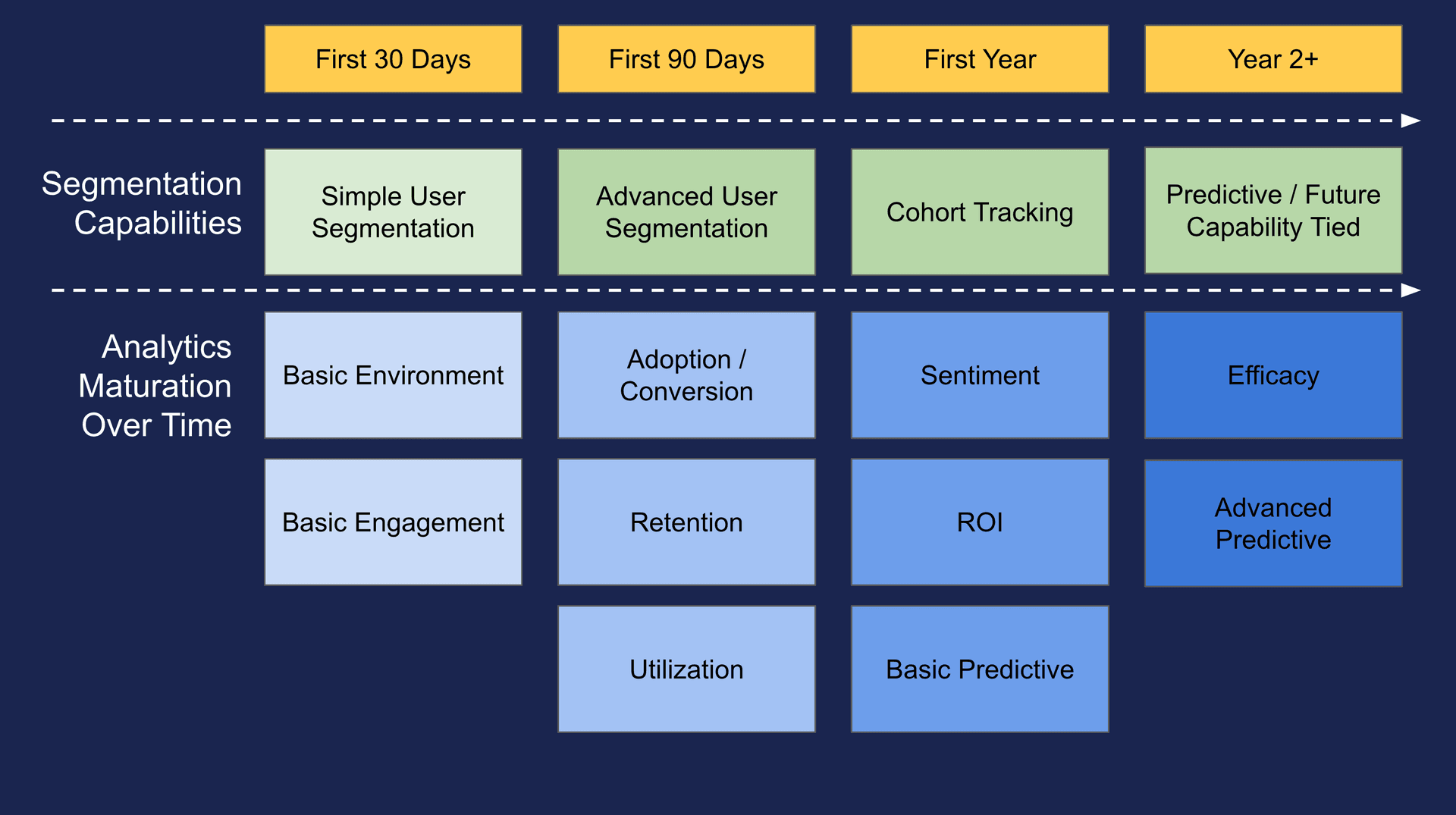
In the First 30 Days
Once data begins streaming into an analytics platform like Pendo, a Product Manager should explore basic, easily actionable analytics efforts. Pendo is designed for Product teams, offering excellent features to start promptly. Within the first month of collecting analytics data, consider setting up the following:
Simple User Segmentation: Your first step in the analytics maturity model is to establish simple user segmentation. Begin by categorizing users based on fundamental criteria such as demographic information, geographical location, or user type. This will provide a foundational understanding of who your users are and how they interact with your product. This will also be a chance early to identify potential additional user metadata that might be needed down the road for more sophisticated segmentation (which will almost certainly be an expectation). Examples of "simple" user segmentation might include new users versus users with accounts > 3 months, or users on mobile versus users on desktop, etc.
Simple User Environment-based Analytics: Next, analyze what your user environments look like, including which browsers, operating systems, and platforms from which your users access your product. Understanding the technical environment in which your product operates can help identify possible usability issues and optimize performance. In Pendo, some of the basic dashboard widgets can be used for this type of insight, such as the Browser Usage or Operating System Usage widgets.
Basic Engagement Behaviors: In the first 30 days, a PM should also focus on analyzing basic user engagement behaviors in their product. PM's should be able to analyze page views and feature usage to get a snapshot of user activity, to answer questions such as, "How many users visit this page?" or, "How much is this feature used on average per week?", etc. This data helps you identify which features are most popular and how users are navigating through your product. In Pendo consider using the Feature Use by Visitor dashboard widget, or the Feature Adoption widget.
In the First 90 Days
As more data is captured and basic reporting is disseminated across the organization, the Product Manager probably will have a good sense of where to focus efforts and iterations with product analytics next. In general, here are some recommendations of where the PM should focus over the first 90 days of their product analytics journey.
More Advanced Segmentation: You should be able to define specific segments of users that matter most to analyze for your product. This probably will get into advanced segmentation efforts that rely on specific user attributes or metadata that you can lean on when defining your segments. Perhaps you might have specific personas of users that leadership might want to understand more fully how they actually behave within your product (typical examples include at-risk or lapsed members, or power users). It is our recommendation that within the first 3 months of product analytics efforts, you will be able to segment your data & reporting to the user groups that strategically matter most.
Adoption / Conversion Analytics: As a PM, understanding whether new users' behaviors within your product experience are expected or not is critical to driving up active user engagement and retention. Similarly, when your team launches new features, it's imperative to know whether users are interacting fully with them or not. So getting up and running with core adoption or conversion based analytics is very important to understand whether your core value proposition for users is hitting home or not. So within the first 90 days of a product analytics journey, we strongly recommend that PM's focus in on those adoption & conversion analytics. In Pendo, consider using the Funnel Report/Widget for conversion based analyses, or the Feature Adoption widget.
Retention Analysis: Once you get advanced user segmentation set up, one of the more critical insights you can report out on is specific user segments' retention over time. PM's who have a solid grasp on retention of various core user segments will be able to more surgically identify opportunities to improve the product experience to ensure these core segments stick around longer within their product. Pendo has a Retention widget and a Retention by Usage widget that should be considered.
Utilization: Utilization is the measure of how frequently and effectively users employ key functionalities within your product. There is a nuanced difference between simple engagement analytics, which reports out on usage of specific features, to a set of analytics insights that reports out on how well or how effectively the product experience is driving engagement across a user base fully. So as a PM, once you get basic reporting of core feature engagement in place, your next maturation is beginning to set up reporting that assesses utilization of those core features.
Within the First Year
In the first year of your Product Analytics journey, expect a familiar pattern: the Product Manager or team will develop increasingly advanced analytics reports, which will be well-received by stakeholders and leadership. However, this will lead to more questions that require further analysis and possibly technical assistance from engineering. This "bringing along" of the organization is a natural progression that a Product Manager should anticipate and be prepared to drive. The Product Analytics Maturity Model we're discussing can help prepare the PM for this journey. Here are some recommendations the PM should consider when planning product analytics efforts in the first year.
Cohort Tracking/Analysis: There will likely be scenarios in PM'ing your product in which you'll want to track cohorts of users over time and analyze various aspects of their behavior to determine whether changes to your product have any longer-lasting impacts that are either desired or not. Cohort analysis relies on the ability within your product analytics capabilities to not just segment users to specific groupings that matter for cohort analysis, but the ability to keep track of them over time against ongoing measures. This is another maturation of your user segmentation capabilities to consider ensuring you have the means to do this within your first year.
Sentiment Analysis: Hopefully you have access to qualitative user data as a PM (typically collected in the form of user research via UX Design in partnership with Product Management, or other sources such as customer support feedback tickets, etc). one of the challenges with these types of primary, qualitative data is that it's hard to link that feedback to specific analytics data within your product. Our recommendation for Product Managers within their first year of their product analytics journey is to find ways to directly tie user sentiment or qualitative feedback, to the specific (actual) activities and behaviors these users exhibit within your product experience. This is harder than it seems! One of the nice advantages that Pendo provides is capabilities that allow Product teams to capture user feedback and sentiment within the product experience, in the form of Feedback and Guides. Because it's all part of the Pendo platform, the users who engage with these feedback mechanisms are also tracked via Pendo, so it's relatively straightforward to correlate qualitative feedback from users with their actual behaviors.
ROI Evaluation: A challenge PMs might face in their product organizations is linking financial data with product data to report on ROI. This typically begins with collaboration between finance and product departments to define investments tied to product roadmaps or revenue from products or features. If these teams can align on mapping data sets for ROI reporting (a significant challenge), the task of building the report can start. Meanwhile, everyone hopes for automated ROI reporting one day. This challenge is common across organizations, so you're not alone if you feel this way. While not easy, we recommend Product Managers pursue this "holy grail" of reporting. Ideally, within the first year, the PM will have provided valuable insights into their products. Equally important is how the PM fosters discussions with these teams and leadership to align on collaborative efforts for true ROI-based reporting. Ultimately, if an organization can see reporting (ideally automated) that links product investments to financial or user engagement benefits, everyone benefits!
Basic Predictive Analytics: Depending on the product you manage, and your organization's needs for forecasting or predicting future behaviors, etc, this may or may not be an interesting or relevant recommendation. Within the first year of capturing rich product analytics data, teams should consider whether they would have a need for establishing any basic predictive analytics based systems that can help inform various organizational departments. Some examples of predictive analytics that teams could consider:
- Predicting revenue impacts based on membership conversion flow optimizations, leveraging historical data that shows conversion #'s to membership revenue.
- Predicting new feature impacts to user engagement based on historical data that shows engagement of similar features.
Beyond the First Year
As a Product Manager aiming to advance product analytics in the second year and beyond, we hope your foundational analytics insights and reporting have been developed and embraced by your organization. If not, we strongly advise prioritizing their establishment. For those fortunate enough to be further along in maturing their product analytics journey, here are some recommendations for the next steps.
Efficacy Measurement: Achieving an advanced level of reporting involves integrating product analytics, financial data, and the efficiency of your product development teams to truly measure your organization's efficacy. If you've already set up automated ROI-based reporting within the first year of your product analytics journey, the next logical step is to incorporate data on product development efficiency. Efficiency should be defined within your organization, often considering metrics based on quality and delivery predictability—key indicators of highly efficient teams. By aligning this efficiency data with your product analytics and financials, you can develop remarkably sophisticated efficacy-based reporting.
Advanced Predictive Analytics: To have infrastructure that supports predictive analytics reporting is a luxury that should not be taken for granted by anyone within your organization. If you do have these infrastructural capabilities, then our strong recommendation as a Product Manager is to continue to build on your predictive analytics capabilities with more and more advanced insights that can truly drive your organization forward.
Every product manager should approach their analytics with the same diligence as they do their products. In other words, we encourage all Product Managers to "product manage" their analytics capabilities.
Conclusion
Every product manager should approach their analytics with the same diligence as they do their products. In other words, we encourage all Product Managers to "product manage" their analytics capabilities. Start with the highest-need, low-hanging fruit insights—those obvious, easiest metrics to track and report on. Then, gradually add layers of sophistication as your organization becomes more data-savvy.
By following this Product Analytics Maturity Model, you can transform your product analytics into a powerful tool for your organization that ties many core functional groups together with leadership.